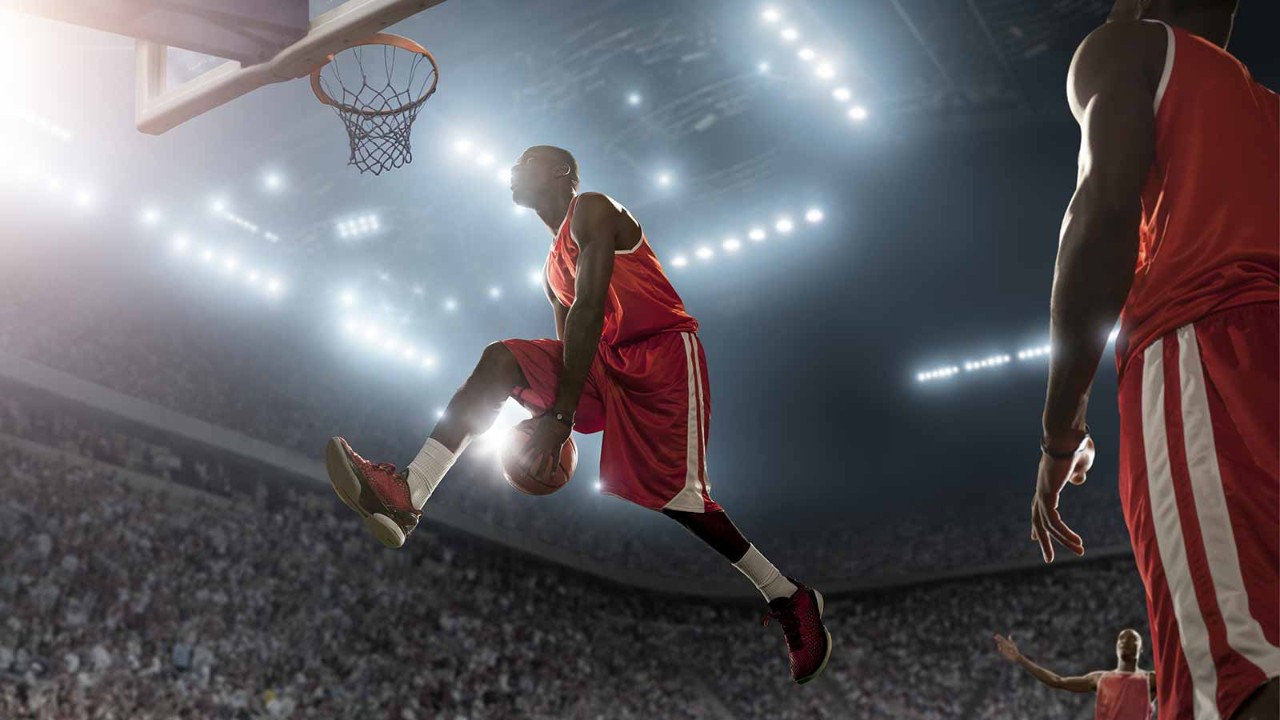
I blame Jack Welch, the former CEO of General Electric. He popularised the use of the Six Sigma process improvement methodology and transformed the bell curve from a niche statistical formula into mainstream parlance. This was not inherently a bad thing, but I think there have been a lot of unintended negative consequences.
Many accounting metrics rely on statistical risk assessment and it’s easy to forget that the underlying models are far from perfect. The bell curve – also known as the normal or gaussian distribution – is probably the most widely used.
It’s an appealing concept, as many phenomena appear to be normally distributed and the maths is fairly simple. Stock option and pension accounting both rely heavily on bell curve maths. The problem, though, is that it doesn’t always work.
The tallest player in the US professional basketball league is 229cm – in theory, a one in 300 trillion occurrence
Behind the curve
Consider height. The average US adult male is 176cm tall, with a standard deviation of 6.8cm. According to the bell curve, there should be about five men in the US who are taller than 213cm (7 feet). Yet the National Basketball Association alone has 23 male players who are over 213cm tall. Admittedly, 12 of them are non-US citizens, but my point is still valid: really tall men are much more common than statistical theory suggests. The tallest player is 229cm (7 feet, 6 inches) – in theory, a one in 300 trillion occurrence.
This has important practical consequences for anyone who is modelling tail risk – ie, the chance of a very rare or ‘black swan’ event occurring. A lot of risk assessment is based on normal distributions, with the result that tail risk is underestimated.
A lot of dumb things were said during the global financial crisis. My personal favourite was the hedge fund manager who described the crisis as a five-sigma event. It wasn’t: his model was wrong.
Every time you see the output from a mathematical model, you should question the underlying assumptions
Shifting sands
Another major problem with normal distributions is that the world is not static. Almost all calculations of mean and standard deviation are based on historical data. This is acceptable when the underlying data, such as male height, changes very slowly. When you start dealing with market risk, using historical data can be much more problematic. For example, every time the economic outlook deteriorates, markets become more volatile because the future has become more uncertain.
Most stock options are priced using the Black–Scholes model, but very few people question the underlying assumptions. This is not an esoteric issue; the cost of granting options can be a material operating expense, so it is important to understand how the cost is calculated. One of the major inputs is share price volatility, which in turn means that the issuing company’s profitability will fall if share prices become more volatile.
Then consider the insurance industry. Extreme weather is becoming more common, so any model based on historical data will underestimate future risk. How can an auditor assess risk provisions when the analysis is based on historical data, which we all know is out of date?
This played out in practice when life expectancy started to increase several decades ago. Defined benefit pension funds went from surplus to deficit over a period of about 10 years simply because all the statistical models were based on backward-looking data.
The answer to this problem is not to seek out better models. The real world has a horrible habit of making modellers look foolish, especially if they are economists.
The real answer is much more mundane. Every time you see the output from a mathematical model, you should question the underlying assumptions. Are they reasonable? What if the future is different?
And never listen to a currently in-vogue management guru.