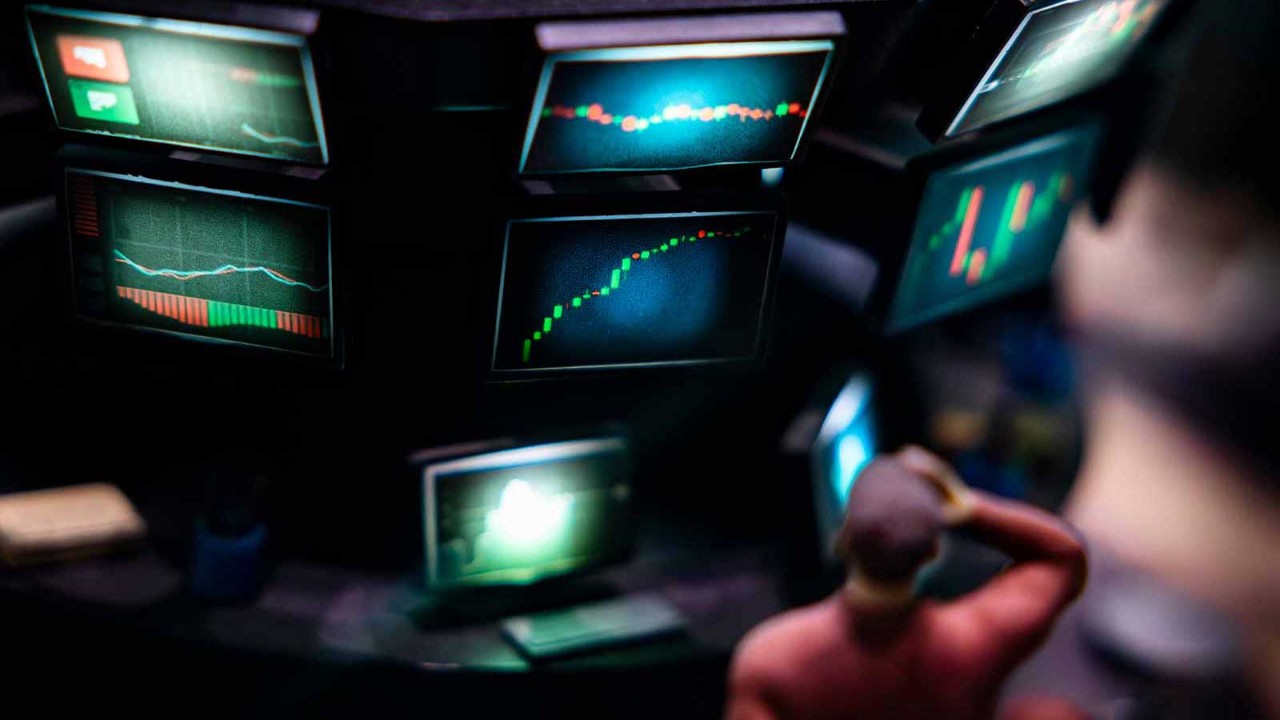
Traditional credit-scoring models, which rely too heavily on historical data, often fail to capture the true potential and unique circumstances of SMEs. This can lead to poor access to credit for these businesses. According to a survey by the Federal Reserve Bank, only 42% of SMEs in the US get their credit and financing needs met.
But now artificial intelligence (AI) could offer a solution. Its advanced algorithms can analyse a wealth of data points that traditional models overlook, potentially enabling fairer, more accurate and more inclusive credit scoring.
The traditional approach often fails to capture the full picture of an SME's creditworthiness
Imperceptible patterns
Traditional credit-scoring models have long relied on a limited set of data points, such as financial statements and credit history. However, this approach often fails to capture the full picture of an SME's creditworthiness, leading to unfair assessments and missed opportunities.
In contrast, AI-powered models use advanced machine-learning algorithms to analyse vast amounts of data to identify patterns that might be imperceptible to human analysts. This data-driven approach allows AI to continuously adapt and refine its credit risk assessments, which could lead to more accurate and reliable predictions.
What is more, AI can also leverage non-traditional data sources, including cashflow items such as customer cash receipts, cash paid to suppliers, proceeds from invoice factoring, trade credit, and other cash receipts and expenses. In analysing this data, AI can better understand the business's liquidity and ability to service debt, providing a more dynamic and up-to-date assessment of credit risk.
By analysing an SME's online presence, engagement and customer sentiment, AI models can gain insights
Another valuable data point is social media presence and reach. By analysing an SME's online presence, engagement and customer sentiment, AI models can gain insights into the business's brand value, customer loyalty and overall market perception – factors that can significantly impact its creditworthiness.
Additionally, AI can integrate other alternative data points, such as supply-chain data, customer reviews and even utility payments, to build a comprehensive understanding of an SME's credit risk profile.
The pros…
AI-driven credit-scoring models could deliver a multitude of benefits for SMEs, lenders and the broader economy. Not only can its more accurate credit risk assessment mitigate potential losses for lenders and prevent deserving SMEs from being unfairly denied access to financing, it can also speed up the credit approval process. Traditional credit-scoring methods are time consuming and resource intensive, but AI automates the process and provides near-real-time assessments, expediting the credit approval process.
AI models are trained on data available in the public domain, but this can lead to false conclusions
It could highlight business loan options that SMEs might have previously overlooked – and expand the range of products available. With a deeper understanding of an SME's circumstances and needs from AI-driven credit scoring, financial institutions can tailor their products and services more effectively.
… and the cons
Despite such promise, some challenges and considerations remain.
AI models are trained on data available in the public domain, but this can lead the technology to make false conclusions and introduce biases and discrimination such as unfair credit decisions that disproportionately impact certain demographics or geographic regions. It seems that human intervention is still needed in testing, monitoring and de-biasing conclusions to ensure that AI-driven credit scoring is fair and free from discrimination.
In addition, AI models are only as good as the data they are trained on. Inaccurate or incomplete data can lead to flawed credit-risk assessments. It’s a minefield that other applications of AI are also struggling to navigate.
And there are issues around compliance procedures. AI protocols need to navigate complex regulations governing data privacy, fair lending practices and the use of alternative data sources.
While the transformative potential of AI in SME credit scoring is undeniable, the above mitigating factors are substantial. But as the technology improves, there is potential to use advanced algorithms, machine learning and alternative data sources to drive more holistic, dynamic and accurate assessments of an SME's creditworthiness.