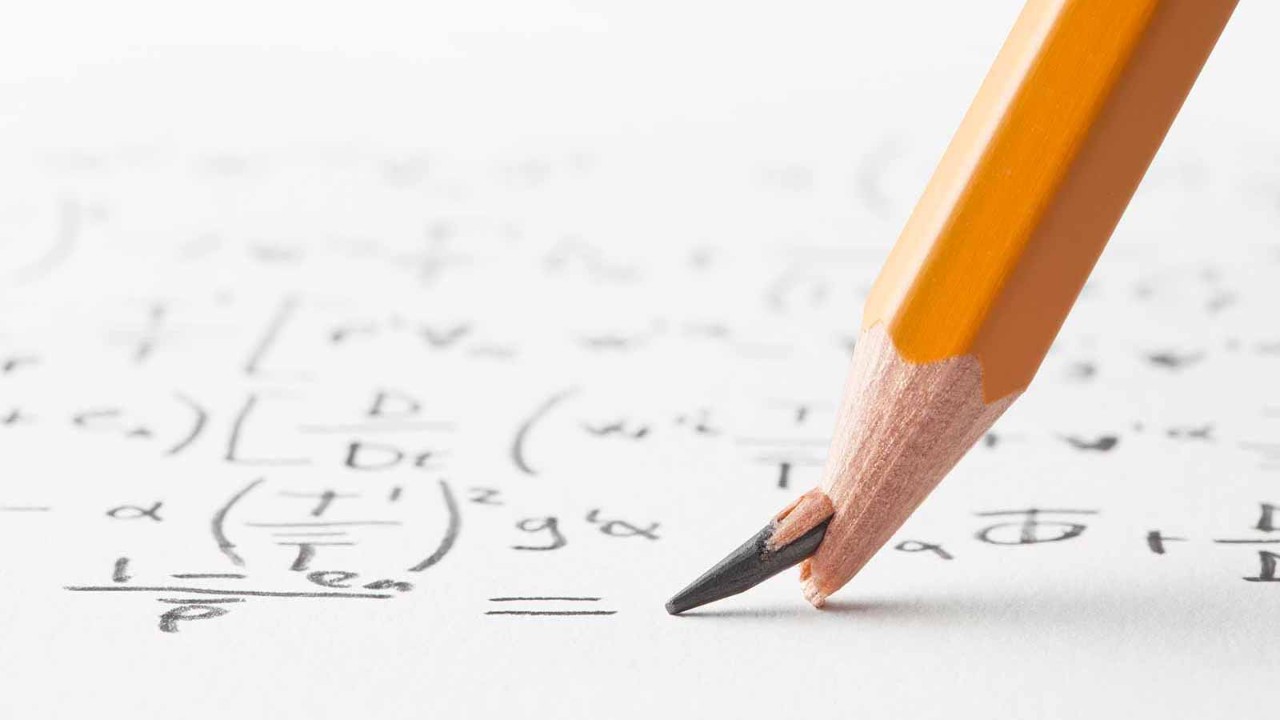
Some words have a resonance that goes beyond their dictionary meaning. ‘Lurking’ means ‘lying hidden as if to ambush’. To me, it sounds even creepier, probably because I read HP Lovecraft’s classic, The Lurker at the Threshold, at an impressionable age. When I first heard the phrase ‘lurking variable’, I just had to know more.
Statistics are omnipresent now. Every day we hear about some new study that claims to demonstrate that condition B is caused by factor A. Very few journalists and presenters come from a statistical background, and boffins like me get upset when a possible link is mispresented as definitive cause and effect.
Most economic models assume that people are rational, despite their refusal to behave rationally
All this might sound arcane, but there is a very important principle at stake here. The entire field of economics – I have always struggled to call it a science – is based on the relationship between different numerical factors. Some of these numbers are real, such as the price of a horror novel; some are not even possible to measure, such as the velocity of money.
At its core, economics is the use of selected numbers (the inputs or variables) to forecast other numbers (the outputs) – even if you can’t measure some of them.
Potential pitfalls
The mathematical process of determining the correlation or interdependence of two or more variables is well established, but there are two huge potential pitfalls.
A lurking variable is a missing input whose absence may cause analysis to be misleading
I have already alluded to the first problem. Most classical economic models assume that people are rational, despite their persistent refusal to behave rationally. Building a robust model that incorporates unpredictable behaviour, such as bank runs or fashion fads, is a task that is unlikely to be completed any time soon. This is one of the reasons why most models fail to spot turning points; the cause of the change is likely to be something you just can’t measure.
There is a second possible problem, which is often overlooked. What if you are measuring the wrong things? What if there is a relevant variable but it’s not in your model?
Too many variables
To illustrate the point, let’s consider Italian life expectancy. An Italian boy born today is expected to live for 84 years: the sixth longest lifespan, not far behind Hong Kong SAR in first place, with 85.3. Italy’s high life expectancy is widely attributed to the Mediterranean diet and lifestyle.
This may be true but it is almost impossible to prove statistically. You can model all sorts of inputs – olive-oil consumption, sunshine, obesity, water quality, unemployment, even happiness – but proving a causal link is elusive. It’s not just that there are too many variables but that some may be missing. Maybe Italians have better genes. Maybe the low birth rate makes adults less stressed.
A lurking variable is defined as a missing input whose absence may cause the analysis to be misleading. The reason why we are inundated with changing dietary advice is that the number of potential variables is almost infinite. You can demonstrate a relationship but it’s another thing altogether to prove that drinking, say, carrot juice makes you live longer.
I would argue that every single model has multiple lurking variables
I think this is one of the reasons why so many economic models perform so poorly. Many are empirical in nature – in other words, we made the formula up but it seems to work – and others have obscure mathematical roots. I would argue that every single model has multiple lurking variables, both obvious and less intuitive.
The next time you are presented with a study that claims to have unearthed a new causal relationship, ask the author if he has considered lurking variables. His answer will tell you a lot about the quality of the analysis.